#47: The Future of Digital Health, Part 2: America’s Data
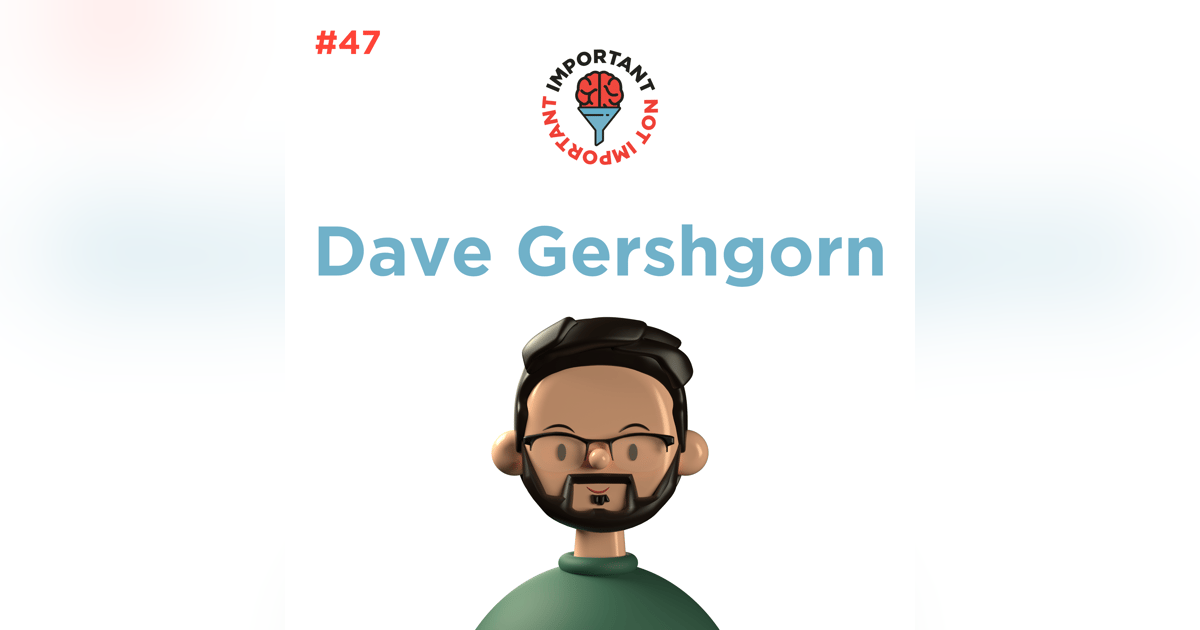
In Episode 47, Quinn goes solo for a minute to discuss: America’s data and the future of digital health. Quinn sits down for a one-on-one chat with Dave Gershgorn, the lead artificial intelligence reporter at Quartz (AKA qz.com), to figure out why our data is different, how the future we were promised is both here and pretty damn far away, and whether/why data is too white. The worlds of healthcare and artificial intelligence are looking – big surprise – really biased right now, but with some effort, we can get to the colorful and diverse future of digital health we talked about with Dr. Indra Joshi and Maxine Mackintosh back in episode 43.
Today, Dave provides some journalist-approved action steps that will help us get a little more informed on the subject so that we can all help make that future our reality.
Want to send us feedback? Tweet us, email us, or leave us a voice message!
Trump’s Book Club: The Life-Changing Magic of Tidying Up: The Japanese Art of Decluttering and Organizing by Marie Kondo
Links: Read Dave’s work: https://qz.com/author/dgershgornqz/
Twitter: @davegershgorn
Dave’s Machine Learning Twitter List: https://twitter.com/davegershgorn/lists/machine-learning
“If AI is going to be the world’s doctor, it needs better textbooks” Machines of Loving Grace: The Quest for Common Ground Between Humans and Robots by John Markoff
AI Now Institute: https://ainowinstitute.org/
Data & Society: https://datasociety.net/
“A Proposal for the Dartmouth Summer Research Project on Artificial Intelligence”
Connect with us:
Subscribe to our newsletter at ImportantNotImportant.com!
Intro/outro by Tim Blane
Follow Quinn: @quinnemmett
Follow Brian: @briancolbertken
Like and share us on Facebook!
Check us on Instagram!
Follow us on Twitter!
Pin us on Pinterest!
Tumble us or whatever the hell you do on Tumblr!
Important, Not Important is produced by Podcast Masters
Quinn: Welcome to Important, Not Important. My name is Quinn Emmett, and Brian has abandoned me once again. Just kidding. Life is complicated, and sometimes you can't make it to your podcast episode.
Quinn: Anyways, we're following up our conversation today with Dr. Indra Joshi and Maxine Macintosh, on the state of artificial intelligence in healthcare today. That one was super fun, and today's is just as great.
Quinn: Today, we're focusing on the data, specifically America's data, why we're different, why the future we were promised is both here and pretty damn far away, and whether and why data is too white.
Quinn: My guest, because again, Brian abandoned me today, is Dave Gershgorn. Dave is the lead artificial intelligence reporter at Quartz, that's QZ.com. He has a lot to say, and is super informed and connected on this stuff. He's also a colleague of Akshat Rathi who has previously talked to us about the state of carbon capture technologies, and whether or not they'll save our collective asses.
Quinn: This is a reminder that the news is super crazy, and we get you. We got you. It feels like the world is going to hell in a hand basket, and I get that, but I've got to tell you there is some hope. There is. You can check out our free weekly newsletter at importantnotimportant.com, where we give you only the latest and the most important news. The big, big, big things that are affecting everyone now or in the next 10 years or so. Climate change, clean energy, cancer, artificial intelligence, antibiotics, space, CRISPR, that is there. We will help you be better informed and feel better about what's going on.
Quinn: All right, let's go talk to Dave. Our guest today is Dave Gershgorn. Together, we're going to talk about why healthcare artificial intelligence is looking, surprise, very, very white. Dave, welcome.
Dave Gershgorn: Thanks so much for having me.
Quinn: For sure, man. Can you tell me real quick who you are and what you do?
Dave Gershgorn: Sure, my name is Dave. I am the artificial intelligence reporter at Quartz. We're an online and technology publication. As an AI reporter, pretty much my job is to stay kind of on top of the latest AI research, but also kind of how AI is being implemented, whether that's in healthcare, in the courts, or automation, or robots, or however it's kind of impacting humans in society.
Quinn: I like it, man. How did you get to AI? What dragged you in this direction?
Dave Gershgorn: Before this job, which I'm super specialized now, I was an editor at Popular Science, the magazine.
Quinn: Love it.
Dave Gershgorn: I was doing a lot of consumer tech stuff on the print side, and then online I was writing about AR and VR, and lasers, which is like the coolest beat on the planet.
Quinn: Sure, who doesn't want to cover lasers?
Dave Gershgorn: Of course. When there's a huge laser and they're going to shoot it, you want to see it.
Quinn: 100%.
Dave Gershgorn: It's awesome. Something I started getting more and more interested in was AI. This was in like 2015, where Facebook was just setting up their research lab. Google was just getting really interested in AI. I kind of saw this formation of an industry, and I thought that it would be really neat to write more and more about it.
Dave Gershgorn: I pitched a bunch of stories to my bosses, and got up on [Jaliway 00:03:41] to cover AI a little more robustly than other people were at the time. Kind of put together what I think are some pretty good covers, that stand up today, on how Google and Facebook think about artificial intelligence. That kind of led into more and more stuff. Then here I am, writing about it full-time.
Quinn: Interesting. You are sort of growing up with the industry a little bit here.
Dave Gershgorn: Yeah, for sure. It's fascinating to see how AI has evolved in the last just four years, since I've been I guess paying attention to it, or three years. The way that I think about it is in 2015, there was still a lot of, "Whizz, bang, look at what AI can do in the industry." Machine learning industry, but also just like Amazon and Facebook, "We can do these amazing new things." Facebook can tags your photos by itself. That's kind of incredible.
Dave Gershgorn: 2016, this was when people started really realizing how much money can be made. A lot of the industry kind of swooped in, and it was sub-reality. 2017, was more of the same, expansion, expansion, expansion, but people were starting to tap the brakes a little bit. 2018, has really been the year where you've seen a lot more consideration of IS and AI, and where these systems don't necessarily fit, and what's really tough for these systems.
Dave Gershgorn: That's what I found fascinating in the last I guess year or so, has been looking at what happens when AI messes up, or goes bad, or is fed the wrong information. Usually, the first two parts of that are born by the third. That's kind of how I think about the progression of AI in the last few years.
Quinn: Right, right. Would you say 2019 is the year where it just all ends? That this is ...
Dave Gershgorn: Yeah, it's all over. I mean if it's not AI, there's so many other things it could be.
Quinn: One of 12 fucking other things.
Dave Gershgorn: Exactly.
Quinn: Probably four of them at the same time. Great, great. Good talk. Super excited about that.
Quinn: Listen, man, as I mentioned a little bit offline, and everybody kind of knows, but just to remind them, the goal with our conversations is to really give people context around these subjects. Pepper you with a bunch of annoying questions, to further flesh it out, and then build just some journalism approved action steps, that in this case people can take to make themselves better informed about AI, and healthcare, and personalized medicine, and all these fun terms that have been thrown at us, but are way more complicated than we're finding out.
Dave Gershgorn: Cool.
Quinn: We'd like to start with one important question, instead of saying, "Tell us your life story," which I just did, I would like to ask you, Dave, why do you feel like you're vital to the survival of the species?
Dave Gershgorn: I think if anyone answers that they are vital to the importance of our species, or the survival of our species or whatever, I think that they're lying to themselves. I think that the work that I do is important. We need as many people as we can asking questions about things that people don't understand.
Dave Gershgorn: If there's one thing that most people don't understand, it's artificial intelligence. All I do is I ask, I have no qualifications or background that really makes me better than anybody else on this topic.
Quinn: Welcome, you're in a safe place.
Dave Gershgorn: I ask really dumb questions of really smart people. I think that if that helps people at the end of the day, then I guess it's a job well done.
Quinn: I think it's immensely valuable. I mean journalism always has been, but in these cases that are moving so quickly, and at the same time hitting some particularly interesting hiccups, theoretically with lives on the line, or say elections, these questions and asking dumbed down fundamental questions, but also ethical questions, is pretty important.
Dave Gershgorn: Totally. I think it's super easy to get lost in the math of it all, without kind of really asking, "Why are we doing this? What is the intention behind automating this specific task over another?" One you get into those intentions, that's where everybody can participate. There are very simple values encoded in those intentions.
Quinn: Sure.
Dave Gershgorn: It's like privacy. It's like as a user of this service, do I want an AI tool? It's like a credit card. They'll give you a credit card. They'll give you an AI tool, because it helps them. That helps their bottom line. It helps the product managers, by pitching AI tools, because they look forward thinking. It helps the engineers making tons and tons of money. It helps the companies looking like they are forward thinking. It's like, do you as a consumer want this AI tool? Sometimes you don't have a choice. Facebook, you can opt out of it, but that doesn't really mean anything long-term.
Quinn: Right, surprise.
Dave Gershgorn: Yeah. It's like kind of a nuanced thing. I think injecting some intentionality into how people think about AI, and even products that they use that have AI in it, can only be a good thing.
Quinn: Sure. We come back to this a lot, and I think we keep coming back to it, but as the great Dr. Ian Malcolm said, "Your scientists were so preoccupied with what they could, they didn't stop to think if they should."
Dave Gershgorn: Totally.
Quinn: Dinosaurs are great, until they're not.
Dave Gershgorn: Yup.
Quinn: All right, Dave. I'm going to blow through just a little context here about what we're talking about here, and why we're talking about it. You can just jump in and tell me everywhere I'm wrong. This won't be as wonky as it could be, or some of ours is sometimes.
Quinn: We're talking about artificial intelligence in healthcare. We've had some conversations about this. We talked to some women in the UK, who work for the NHS, and are heading their efforts in this, which are really interesting, as they are seeking to compile data and start to work on that data, and what roadblocks they're running into, and revelations they're having.
Quinn: Basically, everyone feels like artificial intelligence is the next jam in healthcare. We've got actual hospitals being built, partnerships, public/private partnerships being built, protocols being built, tested, and used in some cases. Competition between AI and doctors in radiology and dermatology. There have been some big, super disappointing, but at the same time, maybe shouldn't have been so disappointing flame outs, like IBM's Watson.
Quinn: Those are the systems, of course, but what about the data? This is a two part dance, right? The way it works, or should work, to take a step back, is you can't just bring in a fresh quote/unquote AI computer machine, plug it into the hospital wall, and boom, it can tell you who's got cancer and who hasn't, or who's going to get it and who doesn't. These things, the algorithms and systems have to have oodles and oodles of training data, that are specific to the topic at hand.
Quinn: Again, side note, generalized AI, the quote/unquote artificial intelligence that can pick out skin cancer, cannot drive your fucking car. That is a long way off. It turns out, it can barely find skin cancer correctly. It needs this training data to sharpen its focus, to find repeatable factors.
Quinn: The problem we're starting to run into, which shouldn't surprise anyone, for a variety of factors, is what if that data is super homogenous? That means if it's tested and trained, and in many cases run on all white people, and you walk in and you're black, or Indian, or Latino, or God forbid, a mix of those things, guess what? It doesn't have a damn clue what to do with you.
Quinn: I think we've seen some of these same hiccups, again, luckily it's pretty early days, in the criminal justice system, even with these new DNA testing kits, 23 and Me, Ancestry.com and such. The data is mostly white European, or sorry, at least they have their roots in white European genomes. That's where the results are headed, or that's where it's most indicative. Healthcare is what we're pinning our hopes on, and it is seen for a long time that this is the stuff of sci-fi, but we're actually told it's not that far out of reach.
Quinn: I go back to our conversation again, and you actually haven't heard this one, because I don't think it's come out by the time we recorded it, Dr. Indra Joshi and almost-Dr. Maxine Macintosh over DNS in the UK. They're working on Alzheimer's and all kinds of things. We've got a long way to go, and a lot of difficult fundamental steps to tackle, like standardizing data, so systems can even begin to read, and parse, and train on it. The problem is, is it's too white.
Quinn: I guess with that, for some context, let's start to dig in here. How do we make healthcare AI training data less white? Let's just do a little more context and perspective, to get into it.
Quinn: Dave, from everything you've covered, and you've done such extensive coverage on this, why is it so white? Why are we where we are now?
Dave Gershgorn: I think that I would say that it's not necessarily completely white. I would say that it's biased on tons of different factors. Each one of those demographic lines splits the data into a smaller, less significant portion.
Dave Gershgorn: You have splits between white, black, Latino, all these different racial demographic blinds, which are more important than things like dermatology and external diagnoses. Then you also have male and female. You have poor and rich. With genomics and the way certain phenotypes are expressed, it's like your environment is super determinant of how you might react to something.
Dave Gershgorn: Whether you speak English or not. One of the stories that I wrote about this, opened up with this startup called Winter Light Labs. They're trying to do this amazing thing where you speak a few sentences, and this algorithm analyzes not what you're saying, but how you're saying it, and how those speech patterns might indicate weaknesses in the muscles of your vocal cords. Those weaknesses in the muscles of your vocal cords can be indicative of Alzheimer's disease, or dementia, or any number of these mental diseases, and you can catch it super early, which is objectively awesome.
Dave Gershgorn: It's a super unobtrusive test. It costs nothing to do. You could do it on an iPad. They're literally doing these on iPads. It's so cool, but it only works if you spoke Canadian English as a first language. When you're a computer scientist and your main goal is to make this thing work, maybe that's not your first thought, but it's a lot harder to have that thought in general, if you don't speak English as a first language, or you understand that your data can be biased.
Dave Gershgorn: I think there's this huge stigma around biased data. It's not the end of the world if it's just a research project, but once you start rolling these things into production, like Winter Light was planning to do, and is testing now, it's like now the stakes are there. Now it's like a serious thing that you're dealing with. This is someone thinking that they don't have dementia, if they do, which that's them not checking a potential tumor, nodule that they find.
Dave Gershgorn: The consequences are huge, and so I don't think it's necessarily just about data being too white. Although, I think that often is probably the case. I think there are tons of other factors. I think it's just like this big idea of bias, and who does your data represent.
Quinn: Let me back up real quick again, just to help everybody really understand how this whole system works from start to finish. Again, I know from self-driving cars, to healthcare, to criminal justice, it's probably coming from a number of different places. Let's talk about healthcare, and you dial it down however you want to find the best example.
Quinn: Let's say you're running a lab, and you're trying to test for skin cancer, or you're trying to build algorithms for skin cancer, or radiology, or again, something like Alzheimer's. Literally, talk to me like I'm making a peanut butter and jelly sandwich here, where do you get data? How does this work? We can't talk about where it's failing and where people aren't getting it, if our listeners don't understand where the hell this actually comes from.
Dave Gershgorn: Totally. From my reporting on research, and talking to a bunch of researchers, what I have seen time and time again is that a lot of this fundamental research, a lot of these companies don't have ... Before they get the VC money, when they're just trying to prove that this is something they can do, you look for anything free that's available. A lot of times this is some data that's made public by a university, some data that's made public by a government, some data that's been made public on a CAGL, CAGEL, whatever, however you pronounce it, competition. Public data that you can use and you don't need to pay someone to put it into production.
Quinn: If I could just stop you. Where does that data come from? Where does a university get their data, or a government, etc.?
Dave Gershgorn: Maybe a government, like the NHS might have it from a trial that they ran. They might have it because ... In other countries, other than the US, if it's a public healthcare system, some of that data might be anonymized and made public in a secure way for the pure purpose of research.
Quinn: Actually, interesting, Dr. Joshi informed us, actually, that the NHS system is actually and has always been for data collection, opt-out. That just couldn't be more different than it is here. I get that, but I'm curious maybe over here, and for everywhere else, I think this helps people really get it.
Dave Gershgorn: Totally, yeah. Universities, people run these trials for either their PHD candidacy, or just medical trials that have been made public for the sake of the public good or other researchers. The ISIC, I believe, is an image collection, it's just a bunch of dermatologists that are trying to research computational ways to solve some problems in the industry. They are collecting any idea that they can find. Whether they had a trial and somebody had a dozen images, they just dump into this database. These images are just basically coming from wherever they can get them. A lot of times, there aren't lines drawn or analyses done, where you can say, "This is 90% male, this is 20% people of a certain skin tone." Because a lot of these are kind of ad hoc and it's whatever data people can get, it's kind of like the lowest common denominator. It's just whatever data is around.
Dave Gershgorn: Now, the bigger kind of industries, industry leaders, they want a pipeline where they can get more data. If you have an app that's going to do dermatology, you're going to capture every single image, and you're going to use that to label through someone who can do it on Amazon Mechanical Turk for super cheap, or pay grad students who actually know what they're doing, super cheap. Then you feed that data back into your system. That's kind of that feedback loop that every AI company kind of aspires towards.
Dave Gershgorn: Once you kind of have something up and running, I think getting that data is a lot easier, but people are typically looking for the most free and adaptable data source that they can find. It's actually interesting, this is kind of what drew me to this topic in general, is where is this data coming from?
Dave Gershgorn: As you alluded to and mentioned with dermatology, in January 2017, the Nature cover story was this group from Stanford who had made an algorithm that beat dermatologists at detecting skin lesions. That got a lot of press. I wrote about it. Everybody else wrote about it. It was this big thing, but I was reading through the paper and I was like, "Where did this data come from?" It came from, I think, 18 sources of data. They had thousands of images, but they had to go to 18 different repositories to find a significant amount of dermatology data. I was like, "This doesn't make any sense. You shouldn't have to do that much legwork for this data."
Dave Gershgorn: I emailed them and I was asking what was the makeup of their data demographically. Did they have people with dark skin? Did they have people with light skin? They said it was predominantly people with lighter skin. There are skin tone gradations, it's the Fitzgerald scale, I believe. It was predominantly people with lighter skin. They had a few, I think, people from India in the data set, so there was some, but it was a vast minority.
Dave Gershgorn: That kind of turned me on to thinking that there's a problem with the way that the data collection happens. The whole thing with selection bias for trials and genomics and everything else, it's basically, "How can we get this data the easiest way, the fastest way, and the cheapest way?" There have been mandates to change that, but I don't think they've really changed much at all. That might be too long of an answer to that question.
Quinn: No, no, no. This is exactly what we're looking for. Just so people understand, these labs that spend 75% of their time fucking applying for grants and not getting them, and are run on shoestring budgets, trying to do this groundbreaking research, are not necessarily painting this for evil, but the setup, the data intake, and that circular system, couldn't be any more different from Google and Facebook, where everyone in the world willingly gives them billions of, not just your data, but thousands of factors of your data, for them to parse for advertisements, and to build their products, and things like that.
Quinn: Those are the two different things, and that's where you get such a wide spectrum, and why they can focus ads so specifically to you, and that cool Marine looking sweater that you imagined in your head once, and now it's in front of you on your Facebook feed. I think there are new, hopefully democratized tools now, when you see the Apple watch, and the iPhone, and suddenly everyone who has an iPhone, which is also a limited subset, but at least a broader subset, can do these Parkinson's trials, or the heart trials with the Apple watch, and things like that. Instead of labs having to pay X amount of money, suddenly it turns out there's a billion IOS devices out there, and they can do these things.
Quinn: You hope that there's progress to be made there, but clearly, we're still hitting some issues.
Dave Gershgorn: I think it's really important to say that nobody is going into this wanting biased data.
Quinn: Of course not.
Dave Gershgorn: There are constraints on how data is collected. There are constraints on what data people can use, and those are legal constraints, financial constraints, time constraints. A lot of this is the function of the system that we live in, but I think this whole overarching theme is this is too important to be slaves to that. I think that it's super important for people to understand this isn't a malicious thing that we're talking about. A lot of the scientists are asking the same questions.
Dave Gershgorn: It's also really important to know that there's dermatology data, there's genomic data, but the kind of data that this machine learning researchers want is very specific. Often, it's not actually what they ... What's out there isn't necessarily what they want. There might be the ISIC database of all these dermatology images, but they're not labeled the right way. I [inaudible 00:24:55] it would have something, like someone draw a line around every single lesion, and it's like a shape factor or whatever, that would tell the algorithm, "This is exactly is where the lesion that you should be looking for," so it doesn't get distracted by other things.
Quinn: Sure.
Dave Gershgorn: That isn't there, because dermatologists don't need that. Human dermatologists just see the thing and they understand, "Okay, this is the lesion and this is the skin around it." When you're talking about very dumb pattern recognition algorithms-
Quinn: Starting from scratch.
Dave Gershgorn: Starting from scratch, they'll find anything. They can be biased by the kind of camera that you use. You know what I mean?
Quinn: Sure.
Dave Gershgorn: When you're cobbling together these large scale databases, with incomplete metadata ... I was talking to the guy who runs the ISIC database, and he was like, "We want to have demographic tags, but right now it's an opt-in situation, for putting any metadata," you know what I mean, "So we don't have the complete thing to give. It's a lot of work for us to give a complete thing." Those are those constraints that I'm talking about.
Dave Gershgorn: Yeah, it's a really fraught, kind of complex thing, where everybody wants something a little bit different from the data.
Quinn: Of course, there's also the natural breakdown, where maybe they don't have purposely, I mean none of these are purposely biased, like you said, or even that accidentally, or maybe not demographically, oftentimes the problem is the data simply, or even the algorithms, reflect the population that suffers from a given ailment, and that's it. They might not have thousands of pictures of healthy skin, which again, I am not qualified to really dig into this, but maybe that's where an algorithm learns how healthy skin goes to not health skin. If you don't have pictures of that, and thousands and thousands of pictures of variations of different skin types and different skin colors, that have been exposed to different sorts of UV, then you're just not going to find those answers.
Dave Gershgorn: Yeah. Just before we get too, too deep into it, there's a definite irony to white guys discussing the bias.
Quinn: That's our whole podcast.
Dave Gershgorn: There definitely needs to be better representation of people having these conversations, and the scientists that are actually doing this work. It shouldn't be a burden on the people of color, the women in machine learning, to have to do all of this work to get themselves recognized by these machines. That's just absurd.
Quinn: It's absurd. The only reason I felt even comfortable inviting you on, is because we'd had a conversation with Dr. Joshi and Maxine Macintosh, two women, and one of whom was in color. We try, we're two white guys. Our guests are over 50% female.
Dave Gershgorn: Totally, I don't mean to impeach [crosstalk 00:27:57]
Quinn: No, no, no. We are happy to be incredibly transparent and forthright, that it needs to be better. Look, Apple ran into this shit too, when they launched their Apple Health King a few years ago, and everyone goes, "There's no way to track your periods in this." It's because the five or six, top six people at the company are over 50 white guys. They haven't thought about a period in 30 years. It's like, yeah, that's going to be a problem. When most of the scientists and doctors are white guys, and that's simplifying it, but not too much, they're just sometimes not inherently going to see that there is a problem in the data, especially if they need it fast and cheap.
Brian: Hey guys, it's Brian, sorry to interrupt. I have a quick favor for you, while Quinn is eating his iced maple scone. Every podcast you listen to begs for a rating and a review on Apple podcasts, and here's why. Not everybody listens on Apple podcasts, of course. You might not be doing it right now. Most of our listeners do, like 70%. Most all podcast listeners are on Apple podcasts. The top charts are a huge source of even more new listeners, and we like new listeners.
Brian: Here's the deal, some weird combination of downloads, and ratings, and reviews, or algorithm, I think that's a word, drive up those top charts. We like being on those top charts, and getting new listeners. We just need your help. If you're listening on Apple podcasts right this second, it's really easy, it'll take between five and ten seconds, max.
Brian: If you're staring at the episodes screen, swipe down. Down at the bottom, there's a little library button. You're going to need to tap that. Then find our show, and then tap that. Scroll down to ratings and reviews, and hit the little buttons, there's little stars. Then there's a little thing that says, "Write a review." You just click that, and then you write a review. Do it right now, we'll wait.
Brian: Oh, that's so nice of you. Thank you for doing that. We love you so much. Okay, back to the episode.
Quinn: Let's talk about the US again, because like you said, some of the more nationally run healthcare systems are in a better place, at least for this front. In the US, do we have a lot of data on healthy people, to be pulled in. How is 10 years of electronic health records going on here? Obviously, those have been very complicated. They're not standardized, yada, yada, but now we've got new systems like Epic coming in. Where is the optimism to start pulling those things in?
Dave Gershgorn: We have an insane amount of health data, but ask Cigna for it, or ask Met Life for it, or ask any of the insurance companies, because they're the ones holding this insane amount of data. We run into a lot of problems when there's a private healthcare system, where everything is segmented because holding that data is a business advantage.
Dave Gershgorn: Recently, when I was talking to the people at Winter Light Labs in Canada, they have all of their health data for Ontario all centralized. There is a organization, uncomfortably named Isis, that is in charge of researching with this health data. You can partner with Isis, and you can use national health data, which is like 30 years of Alzheimer's patients at keystrokes. You can understand how these diseases look in large scale populations. It's just something that the public does not have access to in the United States.
Dave Gershgorn: It's very difficult to get access to population level data for the United States' health, unless you are an insurance company.
Quinn: Yeah, and that's what I'm curious about. Unless you are doing something like starting from scratch, and again, they're not perfect by any fucking stretch of the imagination, but things like they're doing with Stanford doing tests on the iPhone and the Apple watch, and things like that. We've realized, "Wait a minute, we actually have an entire population of people," again, that just use these devices, which is inherently, probably can be a little whiter and more affluent. It's still better than most, and we can do things like motion, and vision, and imagery, and heart rate now, and things like that.
Dave Gershgorn: Even the Google trial that they're running. They are getting, it's like what? 100,000 people that they are paying to be in this trial. It's insane, the level of data that they're collecting on all of these people, but they're going to have probably the largest, highest fidelity source of health data of an enormous cohort, that you could ever imagine. These people are all wearing health trackers. They're getting checkups, and filling out surveys every few days. It's kind of insane the length that Google is going to, and that's just because they have, I guess got a functionally unlimited amount of money to do it.
Dave Gershgorn: If you have a lot of money, then of course there's data. There are so many [inaudible 00:33:14]
Quinn: Which is a huge benefit, because there's nobody better at training these algorithms, especially since they bought Deep Mind a few years ago. What could come of that, and what they've already done with Google Flu, and Project Sunroof, all this different stuff.
Quinn: On the other hand, we look at it and go, "Oh look, it's another huge private company that's going to have all this data. Will they release that? What's the benefit? Will they get into healthcare?" You see Amazon trying to build its own healthcare for all its workers. You just go, "Boy, shit's getting complicated."
Dave Gershgorn: Yeah, and I think that it's going to be crazy. I mean Google's already in healthcare.
Quinn: Oh God, yeah.
Dave Gershgorn: If you see the research papers that they're releasing, in conjunction with Chicago and Stanford, I think, Medical Centers, they are predicting mortality the second that you enter a hospital. They're predicting all of these things, and getting all of that training data. This is how a big company gets training data, they get access to an entire hospital's EMRs for 10 years, and then they train on it.
Dave Gershgorn: They are very firmly in healthcare right now, and they put someone like their top people on it. I think that we're going to see a lot more from Google, going forward.
Quinn: Again, some of that stuff could be incredibly compelling. I've mentioned this before, one of my best friends is working in Southwestern Virginia to try and save healthcare there, focusing on analytics. It's a big, awesome research hospital, but it is in a very rural area, where a lot of people don't take their medicine, they're overweight, they have diabetes, they smoke, etc., etc.
Quinn: Inherently, they go to the emergency room a lot. What 10 years of data from a place like that would look like, with the machines and the money behind something like that, as opposed to him just typing it into a spreadsheet as fast as possible. There are compelling things that could come from that. Sometimes it's going to take these big companies. The question is like, "Do we want to give it to them? Then what are we going to permit them to do with it? What are we going to permit them to share? Are we willing to then let it be public? What's the difference between a corporate company, taking it corporate and then making it public, versus a government doing it?"
Quinn: It does behoove the argument of the UK system of, "Oh, it's just opt-out," and that's it, and that's what it's been. Their key point to me was, and it's a really great listen, is that it has always been opt-out. If we all of a sudden had universal healthcare here, and one of the sticking points was, "Do we make sharing your data opt-in or opt-out," that would be make or break, and we literally would never get the legislation passed. Because it has been that way forever, that's just the standard that everyone is down with, and has been. There's no recent fight about it. It's just like, "This is the way it is." They have all of this.
Quinn: I guess that's a good segway to, what do you feel like are, and again, we'll focus on the US a little bit here, and you can talk about other countries as you're schooled in them, but what are the government roles here? A lot of these are private companies, or the data comes from universities, etc., etc. I do wonder where and how someone like the FDA can help regulate or stipulate more comprehensive data sets, or fund some sort of open source new data drives, to diversify and grow, etc., etc. Could you talk about that a little bit?
Dave Gershgorn: I want to preface this by saying that I don't know every single data initiative that the NIH has.
Quinn: What the hell, man?
Dave Gershgorn: That has ever set up. In the early 90s, the NIH, there was this legislation that kind of mandated the NIH to diversify its trials. This was specifically for things like genomics. Basically, we went from 91% or 96% people of European descent in genomics trials to 81%. It was not hugely successful.There have been these big pushes, and millions of dollars invested in this, but it has not made too much headway, in terms of public funding for this.
Dave Gershgorn: The FDA has actually been very proactive, and a company is working with the FDA to get approval for these AI powered medical devices, have been very proactive. There are two companies right now, I believe I've spoken to both of them, who have FDA approval to market their AI health device.
Dave Gershgorn: The first one was, I believe this company called IDX, and they do this kind of retinal, fund this image scan, that is very similar to what the Deep Mind people are doing with the NHS, because there's very little place for bias to be entered, rather than a camera. They can find a lot from this very simple, non-intrusive image.
Dave Gershgorn: This company has been approved so far, and the FDA has been working for a few years with a bunch of companies, to allow them to market. The way that the FDA works is you have to get approval from them to market your device as a medical device, to doctors, hospitals, the whole nine. Luckily, unlike a lot of American instruments of business, like mergers and whatever, you don't have to run it by the government first, typically. For this one thing you do, which is really good, if there's one thing that you want to have run through the government, it's the healthcare devices.
Quinn: Sure.
Dave Gershgorn: That's why we were able to get so much information off the Apple watch as well, because they had to get FDA clearance. Of course, the FDA clearances are weird and confusing, but at least there's some clarity there.
Quinn: Right.
Dave Gershgorn: These two companies now have FDA clearance, and they're not high stakes things. I think that's on purpose, because these are not like ... It's not going to tell you whether you have cancer or not. I think one is related to diagnosing diabetes, and the other one, it might be looking at bones, to detect fractures and things like that. They're relatively low stakes. I think that these are kind of test balloons, to see how AI systems are operating in the wild. To see how marketing them gets kind of adopted by the marketplace, and all these things.
Dave Gershgorn: There are some regulations, but if you can finagle your app or whatever, that takes a picture of a lesion and suggests you go see a doctor, if you can position it outside of the scope of something like a primary care physician would use, or something that actually makes a health diagnosis, I mean it's kind of the Wild West, as much as anything else is. A health tracker, like Fitbits or whatever, it's a consumer product. The FDA only has so much sway over something, its primary function is to tell you the time, but then it can run your heartbeat, all sorts of things.
Dave Gershgorn: I think that there are some regulations, the more serious it gets. In terms of data sets and what data sets people can use, this is something that kind of people are talking about all over AI. We're so far from legislation on AI training data, or algorithms.
Quinn: What do you mean? I wonder about the other side of that too. Instead of just regulations, which obviously again, are important, we're very lucky that these companies do have to check and make sure things fly, about incentives. Where are the sort of X prizes for data and things like that? Again, of course, this also comes down to then you still have to get people to sign up and to do it, and do devices like the Google devices and Apple devices, and things like that.
Quinn: Again, we keep saying, they are the most personal devices and the most capable devices we've ever had. That is why we keep mentioning them is, is it seems like, at least for now, the lowest common denominator, best opportunity for something like this, where we go, "Hey listen, if we just do this and enough people of enough segments, of which there are so many, opt-in, we could actually have something to build on here."
Quinn: Without knowing variations between populations, and I don't just mean color of your skin or your nationality, but two different people that are from the same country and have the same ancestors, and are even from the same family, could be so different. Without knowing how we all differ, literally down to the genome, we don't have a goddamn clue about what the implications of those billions, maybe trillions of variations are, on any potential current or future treatments. That's where the money is, both figuratively and literally, that's where we start to crack things. That's where we start to find out why immunology cures, literally like 10% of people, does shit for 80%, and kills the other 20%. There is promise there, but we have to start making progress on that data to find out.
Dave Gershgorn: Yeah, and I think that another important thing here is that representation isn't cut and dry. There's no formula for representation. You can talk about, "This data set is representative of New York," or, "It's representative of LA," or, "It's representative of Iowa," but kind of the beauty of AI and the reason why it's so potentially lucrative, and the reason why they want to make these tools, using this software, is because it can be applied at scale anywhere.
Dave Gershgorn: When you're talking about representation in a data set, it's not just like geographic representation anymore. It needs to work, it's really fairness more than representation. There are all these words that kind of mean half the same thing and half something else. Representation is super important. Fairness is super important. It needs to work the same for everyone. That's the overarching theme.
Dave Gershgorn: That something that people try, one way people try pulling the wool over your eyes a little bit, where it's like, "This data set was representative of the population we tested on." It's like that doesn't necessarily mean that it was a fair algorithm that's ready to deploy to everyone. It just means that maybe you curated the tests or validation set, and it matched up pretty well.
Quinn: Sure, and that's again, where I'm happy that they're testing it on broken feet. That's great. I'm glad that we can use those as proving cases, but the next indication needs to be, "We really need to broaden, and diversify, and go deeper here."
Dave Gershgorn: Totally.
Quinn: Dave, your thoughts, again, keeping this objective, more informatory, and empowering yourself to have conversations and understand where we're going and where we are, and the progress we have made. We have made serious progress on this, it's just extremely early days.
Quinn: What do you feel like our listeners can do with, as we like to say, their voice, their vote, and their dollar, to make these data sets better, and to inform themselves, to help build the long road to personalized medicine? Is it conversations with their doctor? Is it supporting specific data collecting efforts, again, something open source or interesting, or something they can just opt into? Is it conversations with their representatives? In each case, from your perspective, what are the things we need to be saying or asking?
Dave Gershgorn: What I have found to be intriguing and powerful is the education of congresspeople in the US on this matter. The other month, Kamala Harris, and Cory Booker, and a few other Senators and congresspeople sent letters to the EEOC, which is the Equal Employment Opportunity Commission, the FBI, I think it was the CIA, and a few other organizations, talking about bias in their algorithms. This is specifically law enforcement and equal opportunity agencies.
Dave Gershgorn: This is something that's very much of the moment. If you can write to your congresspeople and say, "This is an issue that I think you should be on the forefront of," that's going to be huge in pushing this into something that can be legislated, on a common sense basis.
Dave Gershgorn: I think that the number one thing you can do is learn about artificial intelligence, from a way that's not necessarily informed by hype, or the Terminator, or science fiction, but very much in kind of like, not the science of it, but how this stuff functionally makes sense. You need to know what AI is, so when somebody comes at you and says, "We have this new tool that you should try, it's based on machine learning," you're like, "Wait, here are the questions that I could ask, that would let me kind of understand whether this thing is legitimate or not."
Dave Gershgorn: I think probably the number one pitfall that there is right now is just the lack of education. The words machine learning and artificial intelligence are flashy and exciting. It's so varied. It can be a complete farce, depending on who's trying to sell it to you. The literacy in what AI means, where the data comes from, who's backing it, is so, so important. Basically, I think the only way that any of this gets better is that people just know more, and know the questions to ask.
Dave Gershgorn: The number one thing I think that listeners can do is get informed, and read themselves, read for themselves.
Quinn: Aside from your excellent reporting, where are the best places for them to start learning? If you have any thoughts on specific questions they should be asking.
Dave Gershgorn: A book that I found to be immensely helpful with not only understanding AI as it stands, but the history of AI, is the book by John Markhoff, who used to be a staff reporter at The New York Times, now I think he's writing another book. This book is called, Machines Of Loving Grace. It is a fantastic, really well-written history of machine learning, and artificial intelligence, and robotics. I just can't recommend that book enough.
Dave Gershgorn: There are a number of organizations that are interested in AI's impact on society, like AI Now. That's a super interesting organization. Data and Society is a really interesting organization, that I refer to in the story I did on healthcare and AI bias.
Dave Gershgorn: If you really want to learn for yourself, some cool history about AI, read the original proposal for the Dartmouth Conferences. In 1955, they proposed that in eight weeks they could pretty much crack AI, and this was Marvin Minsky, the original AI dudes. They proposed this in 1956, they all got together and they talked about AI, and they thought how they could make these machines with brains, as they were.
Dave Gershgorn: It's kind of one of the first legitimate uses of the word artificial intelligence, but these guys basically made Carnegie Mellon. They made MIT, the AI labs there. They were just like the people. If you read this document, it's just astounding that we're still asking the same questions as they were 60 years ago. It's kind of an incredible piece of history, that you just Google and find online. I'd recommend reading that. That's like fun. I read it like two or three times a year.
Dave Gershgorn: Then, I don't know, follow me on Twitter. Here is also a thing, I have a Twitter list, I think 100 or something people follow, which isn't very many, but those are the most important people on Twitter about AI.
Quinn: That's super helpful.
Dave Gershgorn: I lovingly curate this machine learning Twitter list, and you can just follow it. Then you get 250 plus experts in AI, that I have curated for you, over my years of reporting.
Quinn: Thank you for all that free work. We really appreciate it.
Dave Gershgorn: Of course.
Quinn: That's helpful. I don't think half of our listeners can read or want to read. We have a bunch of nerds.
Dave Gershgorn: Totally, just read tweets.
Quinn: Yeah, just read tweets. It's taking civilization in a great place.
Dave Gershgorn: Yeah.
Quinn: That's super helpful, that's awesome. That's up to the minute, and you can watch the conversation evolve, and you can follow the news, but also analysis, from not just hot takes, but intelligent folks who do the work. That's awesome. We will check that out, and we will follow that as well.
Quinn: All right, listen, we're getting close to time here. I cannot thank you enough for coming and chatting with us.
Dave Gershgorn: Of course.
Quinn: If you have anything you can tell us online here, or you can email later, anyone awesome we should talk to in this field, or any other fields, where people are doing game changing work. Again, we focus on the major issues that are affecting everybody now, or in the next 10, 20 years, again, from space, to cancer, to climate change, to clean energy, to antibiotics, and CRISPR, please let us know. Again, preferably ladies and females of color, to make up for our whiteness and our maleness.
Dave Gershgorn: On AI, especially they show recognition in bias, like the people that are kind of like my North Star, are [Timnet Gebruh 00:51:14] she is at Google.
Quinn: Awesome.
Dave Gershgorn: She also is the co-founder of Black In AI, which is like a group for people of color in artificial intelligence. They are a part of the NIPS conference, which is every December. They are great. Joy Bohemie, whose name I continually mispronounce, but she is at the MIT media lab, and she is fantastic. She does really, really great facial recognition work.
Quinn: Awesome.
Dave Gershgorn: Kate Crawford, a co-founder of AI Now, who is at Microsoft. She is kind of like this top, premier expert and speaker, on the AI and bias. There are so many people. I can tell you more, but those are like the three that I would go to first and foremost, for more information on this.
Quinn: Awesome, yeah, for sure. We will reach out to them. Awesome.
Quinn: Well listen, let's do our last little lightning round here. Dave, when was the first time in your life when you realized you had the power of change or the power to do something meaningful?
Dave Gershgorn: Senior year of high school.
Quinn: Okay, hit me.
Dave Gershgorn: Oh, okay.
Quinn: Yeah, I need an example.
Dave Gershgorn: This is when I first got interested in journalism. I was writing for the school paper. I wanted to write about how the superintendent was taking a pay increase, while slashing teacher salaries. The teacher who ran the paper was like, "I don't have tenure. You can't do that." I was like, "Fuck you, I'm going to do my own paper." I did an underground newspaper, and I wrote my story.
Dave Gershgorn: I wrote all sorts of crazy, silly shit, and people loved it. Me and my friends ... I designed it and got my friends to write for it. We printed 200 copies at Staples. It was like $0.03 extra per copy, and we didn't have enough money to get it automatically stapled, so we sat around stapling all ourselves, and we handed it out in school the next day. People were like, "Holy shit, what is this?" You saw people reading your shit in person, and it was the coolest thing.
Dave Gershgorn: People would talk about it. It was like something that I wrote and made changed someone's mind, nuts.
Quinn: That's awesome, that's super cool. Journalism, it's the best.
Dave Gershgorn: It's pretty rad.
Quinn: We have to keep it alive.
Quinn: Dave, who is someone in your life that's positively impacted your work in the past six months?
Dave Gershgorn: Positively impacted my work in the last six ... I would have to say every reporter that's ever filed a FOIA request. I don't think it's like one person, but I think it's the practice of trying to find information that is not apparent now, is I think something that gets lost in a lot of the daily reporting tasks.
Dave Gershgorn: When I see that on Twitter or through talking with friends, whatever, that just motivates me to do that, which I think more, it needs to be done. I have a project hopefully coming out next year, that will focus on more of that kind of reporting.
Quinn: Awesome, super cool, man.
Quinn: Shit is a little crazy right now, in so many ways. What do you specifically do when you get overwhelmed by all of it?
Dave Gershgorn: I cook.
Quinn: What do you cook?
Dave Gershgorn: I cook all sorts of things. I love to cook. That's been my stress reliever. I cook. You name it. I make a really good sauce. I don't really cook a lot of meat.
Quinn: You don't have like a go to? What's your jam? What are you known for?
Dave Gershgorn: I make a dope ass Sunday gravy.
Quinn: Ooh.
Dave Gershgorn: Like really, really good.
Quinn: Interesting. We might have to get the recipe for that for the show notes.
Dave Gershgorn: There's no recipe, that's the magic of it. It's just like, "What do I have? I've got a can of tomatoes and whatever, and some meat." The rest is just improv.
Quinn: I dig it. That's awesome.
Quinn: Dave, how do you consume the news?
Dave Gershgorn: Poorly and en masse. Mainly, it's a lot of Twitter and RSS feeds, and things like that.
Quinn: So flailing, like the rest of us?
Dave Gershgorn: Exactly. I don't have the solution.
Quinn: Awesome. I don't know who does, besides just signing off forever.
Quinn: I'm going to ask Brian's favorite question, if you could Amazon Prime one book to President Donald Trump, what book would that be?
Dave Gershgorn: A book that I have found a lot of solace in, for some reason, and I know that it would never be read, but I just like to imagine, is Maria Kondo's, The Life Changing Magic of Tidying Up.
Quinn: That would be kind of incredible.
Dave Gershgorn: For some reason, it's like the antithesis of everything that this administration I think has acted on. I think this process of intention, and introspection, and joy, is something that is so sparse everywhere in the news that I see. I would just like to see more of that in the world.
Quinn: It'd kind of be incredible. The whole ethos of the book is basically like put out two shirts and look at the shirt, and if it doesn't immediately bring you joy, get rid of it or give it away. I would just love for a person who has brought literally like the Gilded Age back to the White House, I would love to see how that process goes.
Dave Gershgorn: Totally. Remember in the beginning, I totally forgot about this until just now, it was like the first or second week, and there was this picture of the Resolute Desk and it just had all these papers on it. It was covered in papers, and everyone freaked out, and I think rightfully so. It's like a disrespectful kind of thing, that also indicates that everything is kind of a mess.
Quinn: Who knew?
Dave Gershgorn: Who knew? It was kind of like this amazing symbol. You just got to bring some Marie Kondo into it.
Quinn: I love it, man. I love that book. Somebody stole my copy. I'm coming after them.
Dave Gershgorn: Oh really? I just got a copy to give to my dad for his birthday, and I'm very excited to give it to him.
Quinn: Is he going to appreciate it, or is he going to be like, "What the hell, man?"
Dave Gershgorn: We talked about it, he's going to like it. My parents are in the process of a move.
Quinn: He's ready for it.
Dave Gershgorn: He's so ready.
Quinn: That's awesome. Dave, where do our listeners, where do they stalk you online?
Dave Gershgorn: Dave Gershgorn, G-E-R-S-H-G-O-R-N on Twitter.com. That's really it. I don't really do any ... I don't have a website. It's like three sentences, so don't go there. Just Twitter.
Quinn: Awesome, all right, just Twitter. We will do it. We'll put that ... That list is public, the AI list on Twitter?
Dave Gershgorn: It is public.
Quinn: Awesome.
Dave Gershgorn: If you go to my profile, I think there's like a little arrow button or something somewhere, and you can find my lists. It's literally called Machine Learning. I guess I should also tell you to read QZ.com, where I work.
Quinn: Okay.
Dave Gershgorn: That's a good site.
Quinn: Yeah, we love Quartz here, man. Awesome. Listen, thank you so much for your time today, Dave, and for all that you're doing out there, journalism and looking at the future. You're going to be the Steven Levy of this in 20 years.
Dave Gershgorn: Cool, I don't know.
Quinn: Hey man, hey man, have some confidence here. We need you. You rip on Terminator, but we need you to tell us when Terminator is about to happen. That's going to be important.
Dave Gershgorn: I'll do my best.
Quinn: All right, man. Listen, thank you, and we will talk to you more soon.
Dave Gershgorn: Alrighty, thanks so much.
Quinn: All right, thanks brother. Bye.
Dave Gershgorn: Have a good one, bye.
Quinn: Thanks to our incredible guest today. Thanks to all of you for tuning in. We hope this episode has made your commute, or awesome workout, or dish washing, or fucking dog walking late at night, that much more pleasant.
Quinn: As a reminder, please subscribe to our free email newsletter at importantnotimportant.com. It is all the news most vital to our survival as a species.
Brian: And you can follow us all over the internet. You can find us on Twitter @importantnotimp.
Quinn: It's so weird.
Brian: Also on Facebook and Instagram, @importantnotimportant. Pinterest and Tumblr, the same thing. Check us out, follow us, share us, like us, you know the deal. Please subscribe to our show wherever you listen to things like this. If you're really fucking awesome, rate us on Apple podcasts, keep the lights on. Thanks.
Quinn: Please.
Brian: You can find the show notes from today right in your little podcast player, and at our website, importantnotimportant.com.
Quinn: Thanks to the very awesome Tim Blane for our jamming music, to all of you for listening, and finally, most importantly, to our moms for making us. Have a great day.
Brian: Thanks guys.